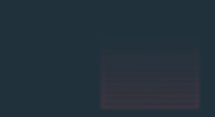
No Limits.
Wix Engineering is the place to learn how to work at scale, to solve robust challenges, and to create the highest quality code. We put a great deal of focus on our engineering culture, continuous learning and personal development.
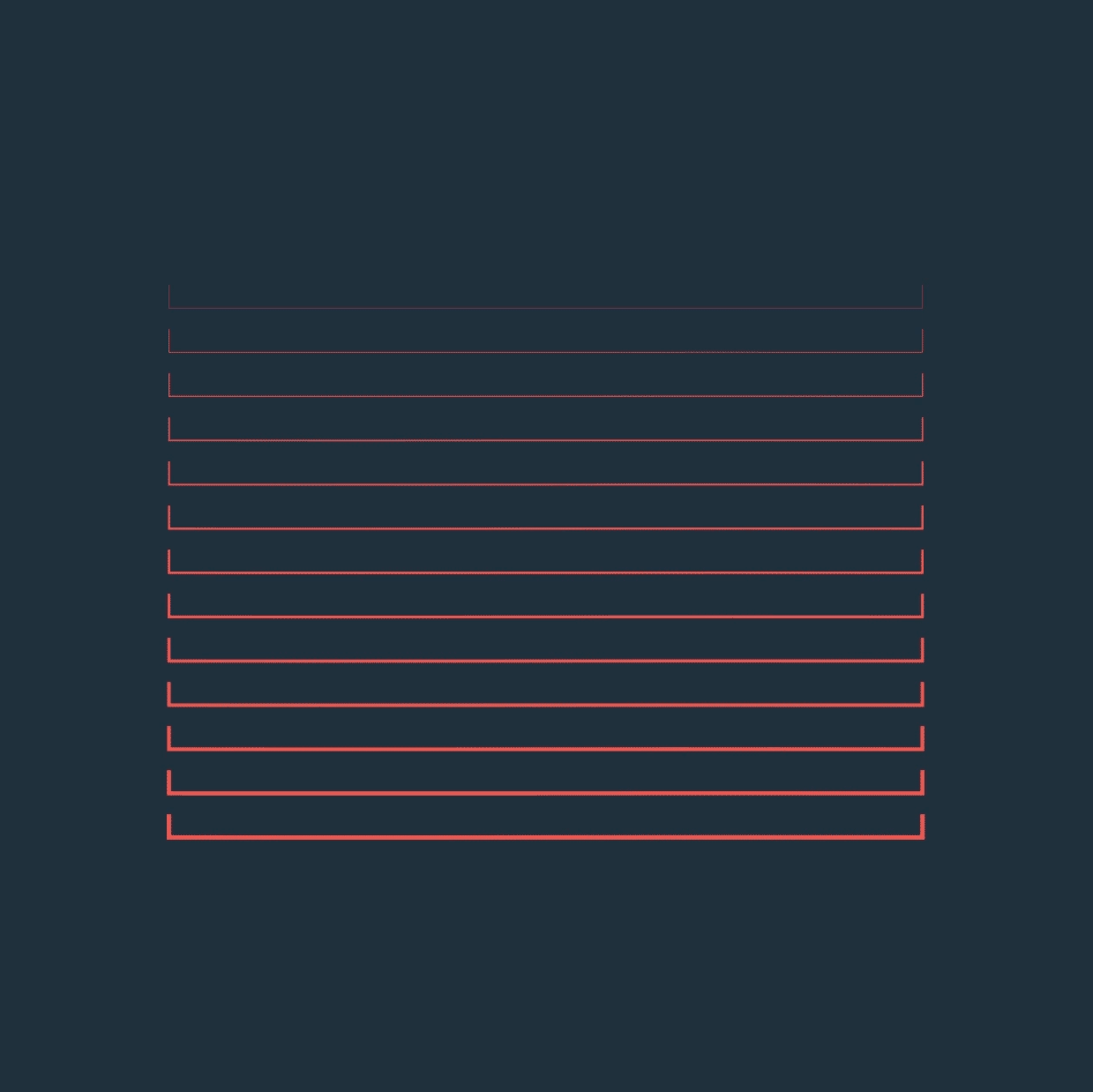
03
Events
Meetups
& Events
Meetups, workshops and other events for the dev community are an inseparable part of how we do things at Wix Engineering. Hosting and knowledge sharing are key to our success as a community. Check out what’s coming up next near you.
05
Students & Grads
Educational
Programs
Wix Engineering is the place to learn how to work at scale, to solve robust challenges, and to create with the highest code quality. We put a great deal of focus on our engineering culture, on continuous learning and personal development. This is the place to start working with some of the best engineers and mentors.

More than 500 millions lines of code.
~250 millions page views on daily average.
~9500 builds a day.
125 600 deployments in production.
Over 20k PODs across all our data centers.
Over 2000 microservices in production.
~1200 created experiments monthly.
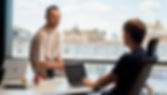
07
Mentorship
Book a Session
We at Wix Engineering love sharing our engineering insights and experiences with the community. We also strive to make the industry a better place, so we offer mentorship and consultation sessions with some of our top engineering minds.
We can talk about anything. From management, software development and scaling to engineering culture, architecture or anything in between.
These sessions are free of charge as a service to the community.
